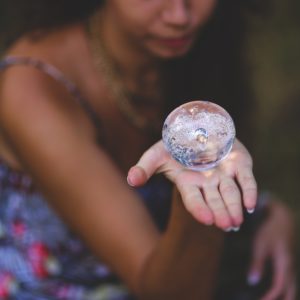
On the eve of the 2016 election, a number of polling websites, including the New York Times, gave Donald Trump less than a 1% chance of beating Hillary Clinton.
In the case of Brexit, it was a hard-learned lesson that “surely not” isn’t an entirely accurate way to forecast whether something will occur or not.
Who else is bored of waiting for China to finish rising?
The problem with predictions is that, by and large, humans are terrible at it. In any scenario, the sheer degree of change and contingency, the volume of variables and the blindness to our own biases prevent us from making objective forecasts. We fail to see that every second of our lives is Schrödinger’s cat playing out a million and one ways.
Experts have been condemned for making predictions at similar accuracy levels as “dart-throwing chimps”. For the most part, predictions are harmless and usually provide good dinner table fodder when we really mess up. The danger arises in when we use our flawed predictions to inform decision making.
The Global Financial Crisis evinces the most prominent example of financial institutions impacted by short-sighted predictions, namely on the invincibility of the real estate bubble and the belief that banks were “too big to fail”. Lehman Brothers paid the ultimate price, and served as a pertinent warning that the experts can be wrong.
Blinded by our biases
So why do we think we are better at guessing what’s going to happen than we really are?
When we guess something right, we pat ourselves on the back, cherry pick our examples and declare it was always ‘obvious’ it would occur. Yet, tweak any of the variables and a completely different scenario will take place. Nobel Laureate Daniel Kahneman explored in the book Thinking Fast and Slow how humans are excellent at pinpointing random incidents from the past, connecting them, and then declaring that whatever eventuated was certain and pre-determined.
We are susceptible to a number of biases and heuristics that prevent us from objectivity and strongly influence our behaviour, such as:
- Confirmation bias – our pesky little habit of selectively choosing information that reinforces our already-formed opinion and ignoring views that argue the opposite;
- Hindsight bias – we think we remember the past as more predictable than it was;
- The anchoring effect – a cognitive bias where we assume an unknown quantity is known to us based on something shown to us before;
- Optimism bias – we are more hopeful for certain outcomes over others;
- Recency bias – we more easily remember and place greater significance on events that happened more recently;
- Salience bias – being influenced by more information that is more obvious or important to us.
Essentially the problem with human predictions boils down to this: we see what we want to see.
That is why the ‘experts’ are shocked when Donald Trump wins his way to the White House, Britain turns its back on its European neighbors and guesses for when China will eclipse the United States as the dominant global power are routinely pushed back.
Computer-powered predictions
We could, however, begin to look to machines, whose deep learning is heralded as a game changer in its ability to forecast future events. Where humans falter, computers can crunch huge amounts of data, process it in almost real-time with an objective* set of rules and pop out patterns and insights that significantly reduce the human biases that have plagued our previous predictions.
Over the last five years, the growth of quantitative-driven investments has grown exponentially, reaching almost USD$1 trillion (with a ‘t’) worth of assets last year. Algorithmic-powered investment strategies are proving to be both more reliable and significantly faster than traditional market analysis. Even Bloomberg news outlet is moving towards utilising machine learning to deliver more accurate and nuanced market predictions with its new Alpaca Forecast AI Prediction Matrix.
On a more micro level, machine learning offers banks the ability to predict in far more nuanced ways that can protect Financial Institutions against traditional lending risks. By processing huge sums of data to identify patterns, algorithms can be used to spot an account closure before it happens, predict the likelihood of a customer defaulting on a loan, and even determine the habits of a customer to make accurate guesses at where they will spend in the future. To add, machine learning used to decipher payment patterns and anomalies could revolutionise financial crime protection.
Removing human interference and relying on quantitative evidence to make better informed decisions is critical to mitigate the traditional risks faced by FIs. When it comes to transforming the financial services industry, the application of machine learning and AI technology is only starting to come to fruition.
I won’t be factitious enough to predict how it will look in 10 years, but I will say I am excited to explore the myriad applications of machine learning in posts to come.
*The term ‘objective’ here comes with a caveat – while machines can apply rules without many of the biases that plague humans, the data used does not speak for itself but is given meaning by humans and programmed by humans. More on this in articles to come!