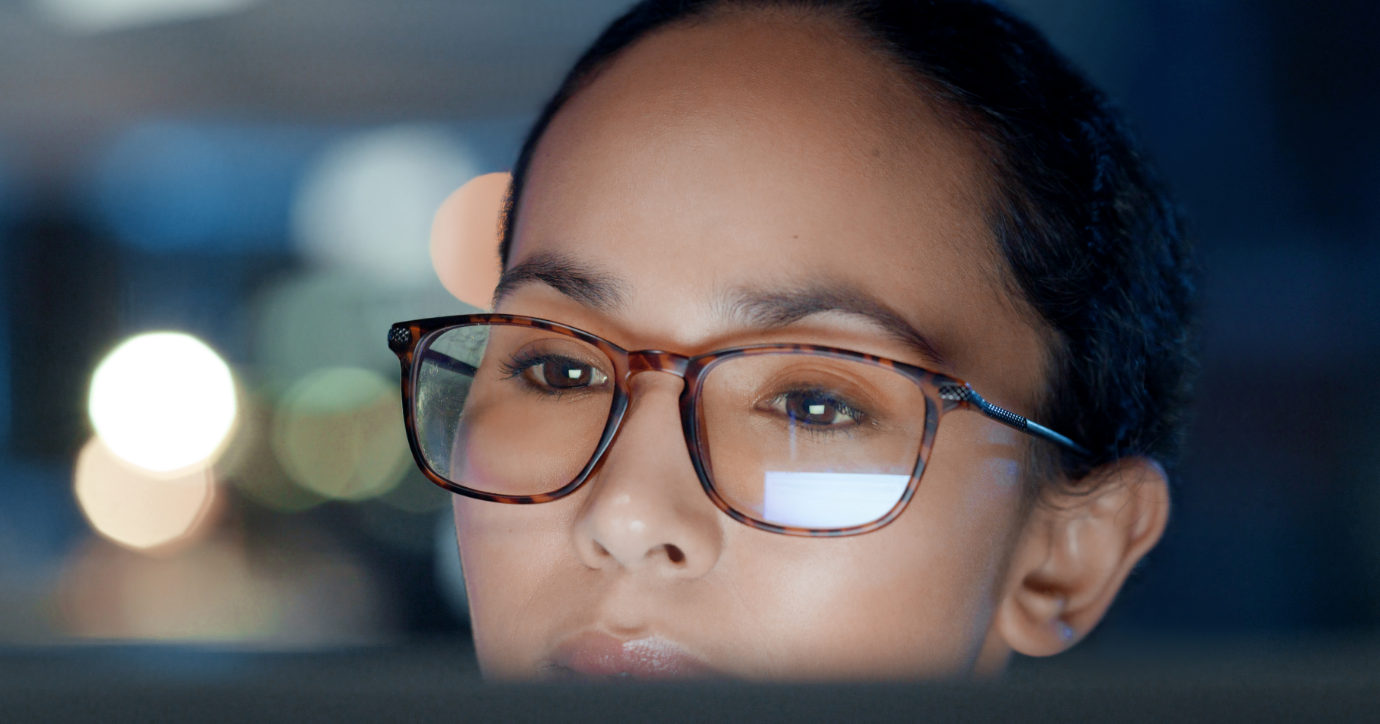
Andrew Morgan, Director of Data at 6point6, believes the data supply chain will make or break any organisation. Here, he highlights the benefits of taking a holistic approach to data entity matching to address the challenges posed by “staggeringly complex” data landscapes.
Empowering business decisions with data entity matching
In today’s digital world, the quality and resilience of data is arguably the most important success factor for making informed business decisions and delivering service excellence. Fast and accurate deduction of valuable insights from raw data enables organisations to address complex challenges and mitigate the risk of inaccurate, potentially damaging outcomes.
Optimising your data supply chain
The process of deducing insights from raw data requires the creation of a data supply chain and comprises 3 stages:
- Creation, capture and collection of raw data
- Transformation and integration of raw data, including entity matching which links records together into a holistic and improved data set
- Consumption and analysis of the final data product
Developing a central data collection strategy is a contemporary best practice to align how teams capture information across an organisation’s processes and transactions. This approach leads to well-structured data sets that support fast and accurate analysis for multiple purposes and reduces the probability of issues such as duplicate records or missing information.
However many organisations, such as central government, have large legacy data sets originating from a multitude of collection systems which each have their own nuances, quality challenges, system update lifecycles and drifting usage patterns. The data is typically gathered from a complex landscape of computer systems, each designed for a single service. This disparity leads to data discrepancies that are hard to identify, trace, or resolve – adding to the risk of data analysis yielding flawed conclusions such as mistaken identity. To appreciate the staggering complexity of such situations, consider that the UK government has provided public access to nearly 25,000 data sets.
Prioritising individuals in your data strategy
Designing services with a user-centred approach prioritises a user’s experience and satisfaction. For example, in the case of public sector services, many government organisations adopt a ‘citizen-first’ philosophy in which citizens’ satisfaction with service delivery is the benchmark of success. This philosophy provides an opportunity to demonstrate operational efficiency and build reputational excellence.
In the case of digital services, users want a seamless and positive online experience. Providing a central public-service portal through which individuals can manage their current and historic data such as driving licences, passports or medical service providers, is a significant convenience both in terms of access and reusing information for new transactions.
Security and privacy are paramount and data protection laws will apply to processing of personal data. Incorrectly attributing data records to a citizen or failing to associate a record with a citizen is at best inconvenient and could have damaging consequences, leading to low user satisfaction and reputational risks to parties involved with developing and operating the service.
These expectations of service necessitate strict data governance and data sharing across departments. However, the complexities and disparate considerations involved in bringing a myriad of different data sets together should not be underestimated. Reliably creating a single view of citizen transactions across public sector services is a critical challenge. That’s why government departments and agencies increasingly value trusted data specialists to develop optimal solutions.
Integrating raw data with data entity matching
Data entity matching is a powerful approach you can use in the second stage of the data supply chain to deal with disparate raw data sources. With this approach, you can interrogate multiple data stores to identify records that most likely relate to an entity of interest – an entity is a unique object that data science tools can process as a single data unit, such as a person, school, product, invoice or chemical.
Methods of data entity matching are evolving as technology progresses. Simple rules-based matching is one basic method, however this method will not readily differentiate between true positives (records correctly attributed to an entity) and false positives (records incorrectly attributed to an entity). For large data sets, the level of uncertainty in the results can rule the sole use of this method out.
Machine learning approaches use contextual information to achieve better accuracy and efficiency. For example, when trying to entity match records associated with differing names (Janine Smith, Jan Smith, J. Smith) to an individual, AI can identify a name’s language of origin and know how it may vary within that language or culture, as well as other identification fields such as dates of birth and addresses.
Machine learning approaches also enable matching names across languages. For example, performing checks for security clearance could require entity matching of records where the individual’s name is in a different language or script. In addition to using text, data entity matching increasingly uses biometric recognition.
Current data matching software offers a tailored approach and high accuracy
The optimal data entity matching approach depends on both the data sources and the project objectives. Developing a tailored and overarching plan that embraces the sources, integration and future exploitation of data is the key to success.
Automating the complex task of data matching clearly offers many benefits such as fast processing of large amounts of data. However, human intervention is still required to investigate uncertain matches. It is the accuracy of the entity matching software, which is crucial to reducing the number of records requiring human review.
Systems such as Rosette® by Babel Street combine human knowledge built into artificial intelligence (AI) and more than a dozen specific linguistic algorithms — such as phonetics, transliteration spelling variations, out-of-order names, and nicknames — to provide a fast and smart matching system that interprets and analyses data from many different perspectives. For every comparison, Rosette produces a match confidence score and an explanation of what factors went into the calculation. This explainable AI enables users to significantly scale and fine-tune the system’s match behaviour to reflect the specific nature of the data, language variations and operational priorities of an organisation. This agility and targeting helps to optimise outcome accuracy and minimise false positives and false negatives (records that should be attributed to an entity but are not).
Other types of system focus on implementing specialised algorithms to do probabilistic record linking. Often these types of systems will use the Fellegi-Sunter matching algorithm which provides users with a probabilistic match which is explainable. The Ministry of Justice has open sourced an implementation of this system.
Both systems use score thresholds that indicate confidence in the matches, so users can validate and tune the record matching to a threshold that provides trusted linking of records. For example, a score higher than 84% would be considered a match, whereas a score lower than 76% would not, and a set of human testers would investigate results that fall between these thresholds during the validation phase, to review exactly the cutoff that maximises the records linked but minimises errors in linking.
Once records have been linked and integrated, these systems allow the most highly complex and labour-intensive searches and assessments of names, addresses and dates to be automated and undertaken in real-time. The time-saving benefits from such automation, accuracy and reliability are considerable. The immediacy of insight also facilitates more informed decision-making and can prevent wrongdoing such as fraudulent activity.
Conclusion
Organisations need high-quality data supply chains to operate effectively in today’s business environments and to be ready to act on new opportunities. Data supply chains often directly determine the standard of service that an organisation provides and supports optimal, timely business decisions. They also drive holistic operational efficiencies and mitigate risks which could cascade and impact services.
Data entity matching is a crucial component of a data strategy for all organisations that process large, disparate data sets. It enhances the data collection strategy by allowing organisations to harvest data from many different sources to construct and act on the bigger picture. By linking the records together effectively, organisations are able to better observe a 360 degree view of their customers in the wider world they operate in, offering significant benefits for transforming the way your organisation works.
Even with sufficient staffing and funding available, it’s often harder than expected to implement these data entity matching systems. The technical challenges are not the only difficulty, as the change approach and smooth transition to new processes can also be difficult to plan and implement. Working with a trusted partner – who understands how to develop holistic data strategies and optimise data entity matching tools – can bring your ‘citizen-first’ vision to life.
About Babel Street
Babel Street is the trusted technology partner for the world’s most advanced identity intelligence and risk operations. The Babel Street Insights platform delivers advanced AI and data analytics solutions to close the Risk-Confidence Gap.
For more information, visit babelstreet.com.
Andrew Morgan
Andrew Morgan is the Director of Data at 6point6. He is the author of “Mastering Spark for Data Science”, a text book for learning Scalable Data Science, and has also published several open source tools. With over 25 years of data projects behind him, Andrew now draws on his deeply technical experience to manage and lead high performing data teams.