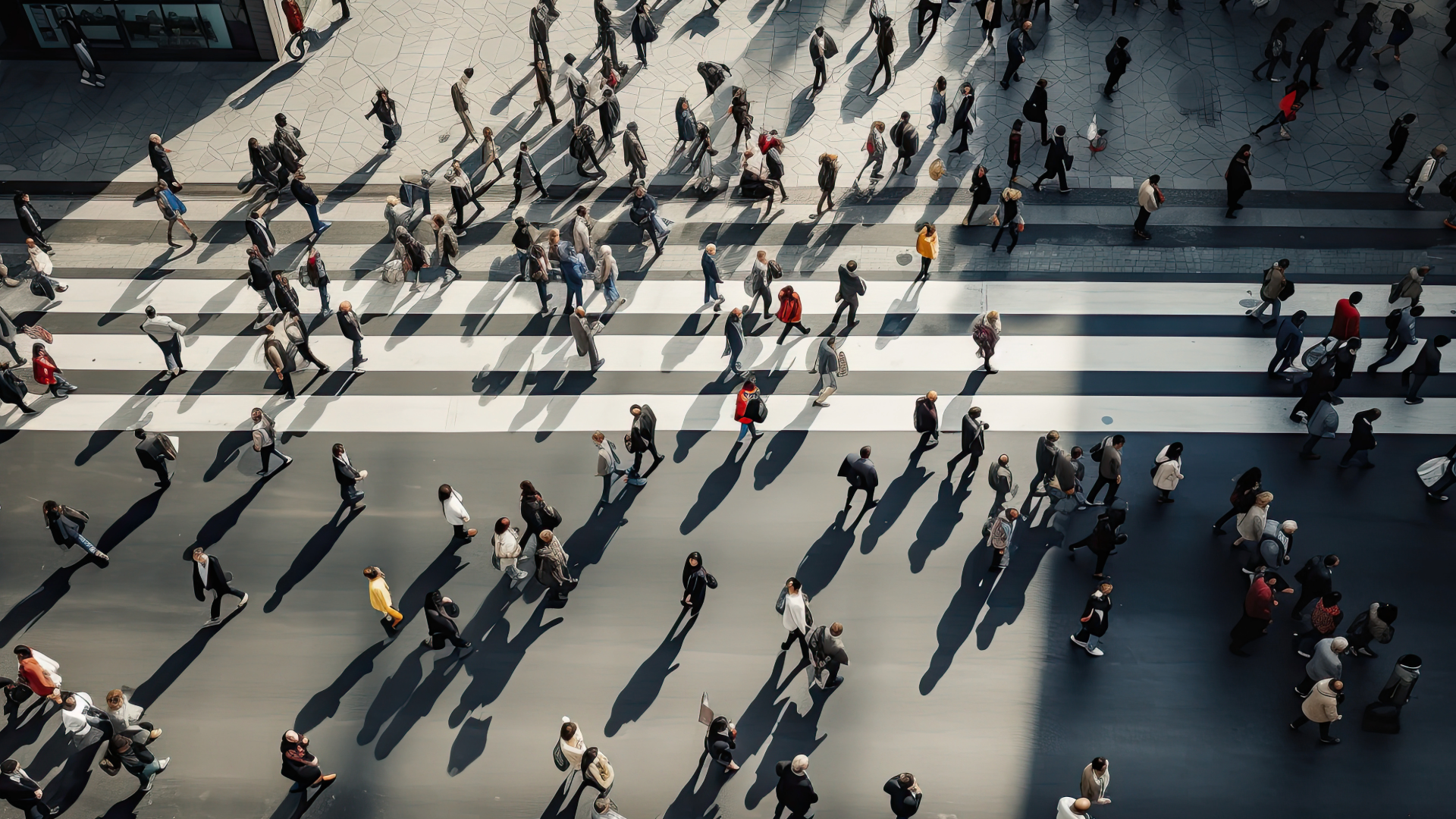
Artificial intelligence covers a broad spectrum of computing, including knowledge graphs, often classed as narrow AI. In this article, we explore how knowledge graphs can assist with pensions dashboards and improve your ability to automatically complete tasks that have been previously offshored, such as manual identity matching.
Pensions dashboards will be a vital tool to help savers plan for their retirement; however, implementing them across the industry has been difficult. With another postponement of the Pensions Dashboard Programme announced in Parliament, integrated service providers (ISPs) and pension providers have been given an opportunity to address this project's many challenges. While we await a new date for the Pensions Dashboards Programme launch to be announced before 22 July 2023, affected firms should not rest on their laurels.
The greatest challenge facing pension providers when responding to pensions dashboard requests is data matching: ensuring the record you hold matches the person enquiring. The customer may have moved address, got married, or a past employer may have misspelt their name. However, the opportunities for mistakes in the core identity attributes of a person may be limitless but not impossible to resolve.
Pensions dashboards will only verify first name, last name, date of birth and postcode; if the data matches, you will respond to the dashboard with a “Possible Match”. However, if you only have a “Partial Match” for the customer, you must instruct the customer to contact your helpdesk. So it's in your own best interest to cleanse the data, remove duplications and resolve unmatched identities before pensions dashboards come into service. Not paying your ISP to duplicate records is an additional benefit of this cleansing.
A well-designed person-matching service, using technology that leverages AI to help cleanse your dataset and resolve possible matches, can help reduce the administrative overhead. This will also increase the probability of returning a correct match to the pension dashboard.
Our recommended approach to enhance your existing person-matching service is to implement a knowledge graph . Much in the same way as social media and marketing companies use knowledge graphs to link relationships with your friends, family and products you may like to purchase, you can construct a knowledge graph to help associate identity attributes to a person. Knowledge graphs also provide the hidden power of predicting new relationships. This is why knowledge graphs power social media companies.
Knowledge graphs offer the ability to build a representation of a person in a way that’s easy to visualise and understand. They include a person’s details (known as attributes), such as their name, date of birth, and postcode. Knowledge graphs allow us to describe the relationship between “Name” and “Postcode” while rating the strength of the relationship. For example, “Name” and “Postcode” may have a strong relationship (10 out of 10), as the customer has previously responded to a letter, so you know they are highly likely to live at that address. By building a network of relationships between a person and their identity attributes, we can accurately say that two separate records belong to one person and conversely, two similar records belong to two separate people.
With enough data, we can also use ‘link-prediction’ to predict certain things about the person, which we can later verify, for example their correct address and investment preferences etc. Link-prediction helps us predict unobserved links within a knowledge graph, for example social media companies use link-prediction to successfully predict other friends a user might want to connect with and many knowledge graph databases now have built-in prediction/recommendation-engines specifically for this.
An added benefit of knowledge graph databases is that they are quick to build and easy to understand by developers and engineers. Their flexible data schemas (often schemaless) make it easy to adapt to your evolving business requirements. They also assist in the deduplication of records, and some knowledge graphs now come with built-in fuzzy matching that further enhances their ability to match records.
They are incredibly fast and frequently offer robust enterprise features, including security and scalability. At 6point6, we predict that knowledge graphs will quickly start to replace traditional fixed schema databases.
Figure 1. Basic graph concept of an Identity problem in a Knowledge Graph - is J Bloggs also Joseph Bloggs?
Your knowledge graph powered identity service will traverse the knowledge graph to resolve an uncertain identity. It will do this by identifying similar neighbouring nodes to a target node, i.e. a person who shares a similar name at similar physical and email address with another person. Using a mix of Natural Language Processing (NLP) techniques, statistical methods and traversing these nodes to identify other similar connected nodes and their adjacent nodes, the scored similarities are ranked and weighted to establish if a match exists or not with accuracy.
With over ten years of developing services for data matching people with government customers, financial services and pension companies, 6point6 is ideally placed to help you investigate and implement the right AI solution for business-as-usual functions. If you already have an operational matching engine, 6point6 offers an independent assessment service to establish how effectively you will match records based on pension dashboard requests.
For further information on how we can support your organisation, contact us at enquiries@6point6.co.uk.
ABOUT THE AUTHORS
Martin Jordan leads 6point6's Financial Services and Commercial practice, specialising in designing and building complex secure services, including the authentication service for UK Immigration services and numerous knowledge graph solutions in Financial Services.
Joe Hearnshaw is a Lead Data Engineer, who played a pivotal role in developing one of the pioneering COVID-19 Human Knowledge Graphs in collaboration with NASA GeneLabs and UC-Berkeley.